In my previous post I gave hints how one can estimate the quality of a player as a batsman, bowler or all-rounder. The idea is rather straightforward. Good batsmen will increase their cumulative runs at a much faster pace as a function of matches played, while bowler will increase their cumulative wickets at much faster pace.
The Criterion
So I looked into the data of about 400 players, for their total runs and wickets over their career. The plot is shown below. In panels A runs are plotted as a function of matches played. It turns out that the top 20% batsmen in the history of test cricket increased their runs at nearly 72 runs per match. Top 20% bowlers on the other hand increased their runs at about 10 runs per match.
Similar trend is bowling data, where top 20% bowlers increase their wickets at 4.73 per match.
So 72 runs per match and 4.7 wickets is a criteria to be a good bowler or batsman, respectively.
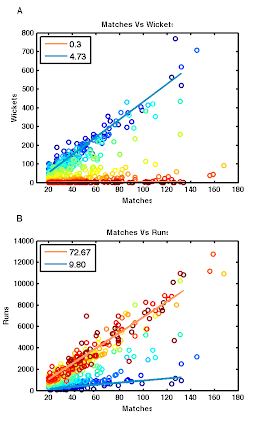
What is an all-rounder
These two numbers (72 for batting and 4.7 for bowling) also can allow us to suggest how close someone is close to be a good bowler and batsman simultaneously i.e. all-rounder.
How do we check whether a player has been a more of a bowler or a batsman or both. In fact, by following careers of some players, like Steve Waugh who was considered as an all-rounder in his early days but ended his career as a batsman. Similarly Ravi Shastri. That is a player with good abilities with bat and ball, can switch roles very dynamically.
So I thought to see the runs per match (run slope) and wickets per match (wicket slope), over a period of 10 matches. This window of 10 matches was slided by 3 matches to get another estimate. The sliding can also be done with 5 matches as many Test series are of that length, but then I consider the fact that an allrounder may not play full series due to injuries or such factors.
With a window 10 matches which was slided by 3 matches, I calculated the run-slope and wicket-slope of 28 players. Average run-slope and average wicket-slope are plotted across each other. The players name is indicated in the figure.
It is clear that Brian Lara, Ricky Ponting, Rahul Dravid, Sachin Tendulkar are right on the top, together with Sunny Gavaskar. This kind of plot also reveal similarity between Viv Richards, Alan Border and Javed Miandad.
On the bowling side, that is in left-bottom corner, all usual suspects (Glen McGrath,Ambrose, Warne, Murali) are placed.
Now the allrounders are in the middle of this plot, the likes of Imaran, Kapil, Botham, Daniel Vettori, Flintoff. I also added Vinno Mankad and Keith Miller to get a historical perspective.
So indeed the good allrounders are those who maintain a good run-slope and wicket slope.
What wait, what about Gary Sobers and Jacque Kallis?
The curious case of Sobers and Kallis
In figure above Gary Sobers is placed slightly higher than Jacque Kallis. Both are great players and simple analysis indicates that Kallis is slightly better than Sobers. But in the figure above, subtle differences are accounted for and we see that Sobers was slightly better, both as a bowler and a batsman.
To get a detailed look on this, I plotted the progression their cumulative wickets and cumulative runs in figure below. The red trace(Sobers) in both panels (A,B) is above Kallis (blue trace).
Panels C and D show the run-slope and wicket slope for both in 10 match segments. An interesting picture emerges. Sobers leads Kallis on batting scale very early. Actually its the inning of 365 runs that gave Sobers an early surge in run-slope. Kallis was a bit slow in scoring runs early on. Both players did good with ball only in their mid-career i.e. around 40-60th matches and that improved their image as all-rounders.
From panels C and D in figure above we can safely conclude that in their mid-career both Kallis and Sobers were highly comparable, in fact, tended to be more like bowling all-rounders . Sobers started to be more of a bowler towards the end of his career as see by dip in red trace in panel D. Kallis is having a very good time with bat but struggling with ball of late.
In general both Sobers and Kallis more or less all the time were in top 20% batter group, but only occasionally made it to 20% bowlers.
These dynamic changes in the nature of performances over short periods of 10 matches in case of Kallis and Sobers prompted me to do make similar figures for Ian Botham, Imran Khan, Kapil Dev and Richard Hadlee.
In the beginning of their respective career the four celebrated all-rounders were more or less alike, but then around 50th match Richard Hadlee outperforms his compatriots in batting!!. Just like the beginning all four all-rounders had similar decline in their batting and bowling towards the end. Kapil Dev observed a slower decline, which perhaps is the reason of his longevity.
Again these four all-rounders were nearly always in top 20% bowlers but only occasionally in top 20% batsmen.
From an all-rounder to batsmen
Further, I wanted to look at the career progression of Steve Waugh, Ravi Shastri and Sanath Jayasuriya. Steve Waugh was regarded as an all-rounder in the beginning. The analysis show that he had a start like that of Kallis and Sobers but he was far from the class of Imran/Botham/Kapil. His decline as a bowler and rise as a batsman is clearly visible in the red traces in panels C,D below. Towards the end he was a batsman, but you don't need to to bowl when Glen McGrath and Shane Warne are on your side.
Similarly Ravi Shastri was a bowler of about average class in the beginning and ended as an average batsman and a poor bowler.
Sanath Jayasuriya has interesting spell in his career when he was a good bowler, or a good batsman or both. Around the time of his 50th test match he was in the class of Sobers/Kallis, currently he is just a good batsmen. They way he is showing no signs of retirement, who know he may again return to his all-roudner status for a short time.
In all I think estimation of run-slope and wicket-slope in short duration is a very good indicator of the abilities of a player as an allrounder or batsman or a bowler.
This analysis reveals that the four celebrated all rounders (Kapil/Imran/Botham/Hadlee) were in fact more bowlers who were very effective with bat. On the other hand Sobers and Kallis are more of batsmen who are really good with ball.
right arm over
Arvie
PS: In the plots above I should have added the error-bars but I just wanted to keep the figures uncluttered, but if someone needs I can provide those.